I spent $343 for one month of blueprint. this post shares what I got and what my thoughts on it are!
overall I’m a fan of most ingredients and the overall composition. I came up with many of them on my own a few years ago, many of which are listed here. the doses are pretty reasonable and I trust the sourcing to at least be above average
this post is not sponsored or paid for in any way, but I’ve met BJ and think he is reasonably smart and funny. below I include images, nutritional information, and plenty of links and side notes to share what I’ve learned!
Apr 13 2024 edit: added response comments from Blueprint: ctrl+F “BP” to find them
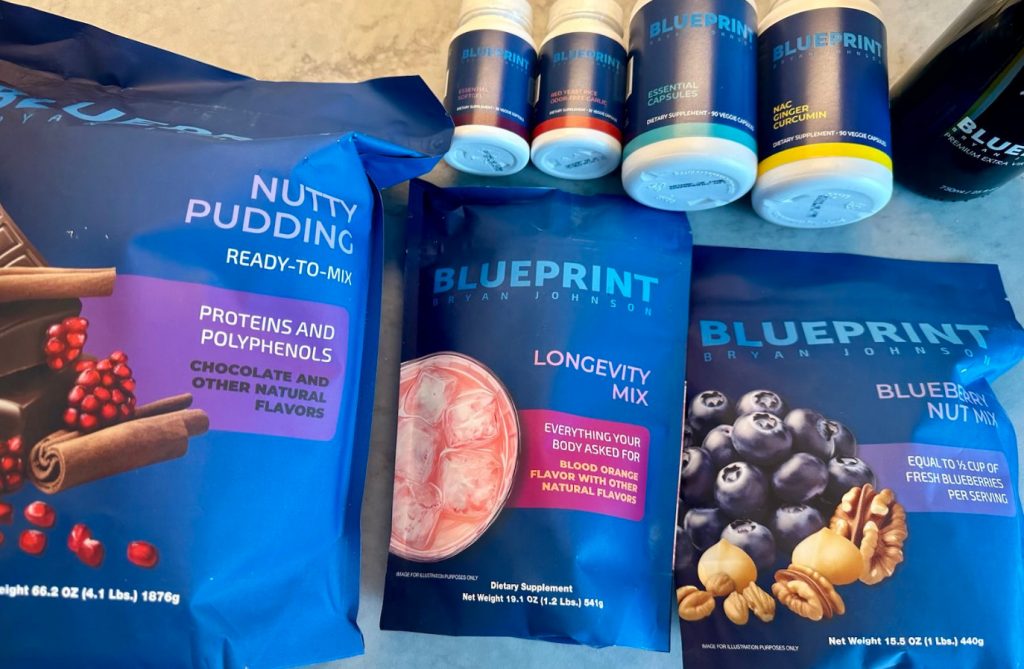
Nutty pudding
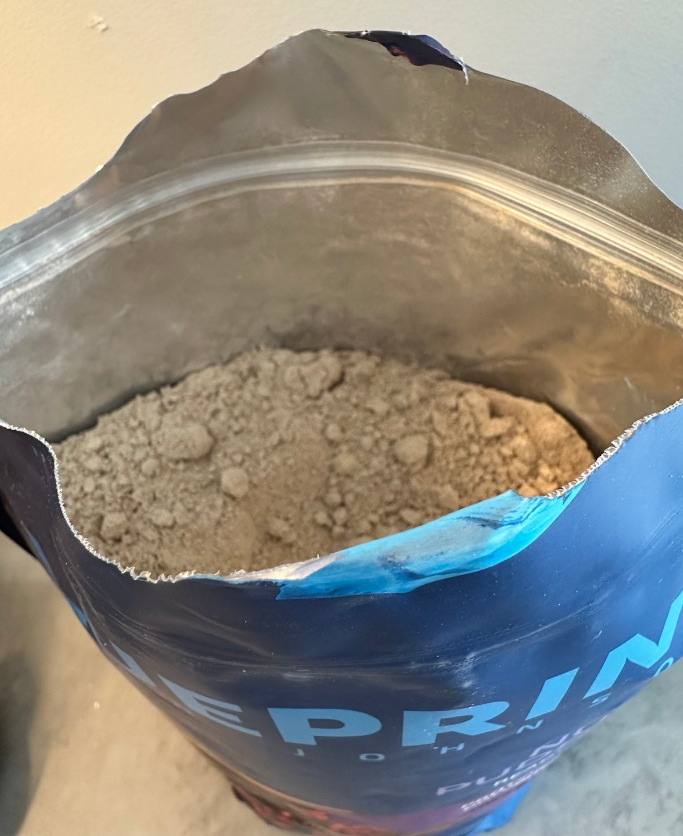
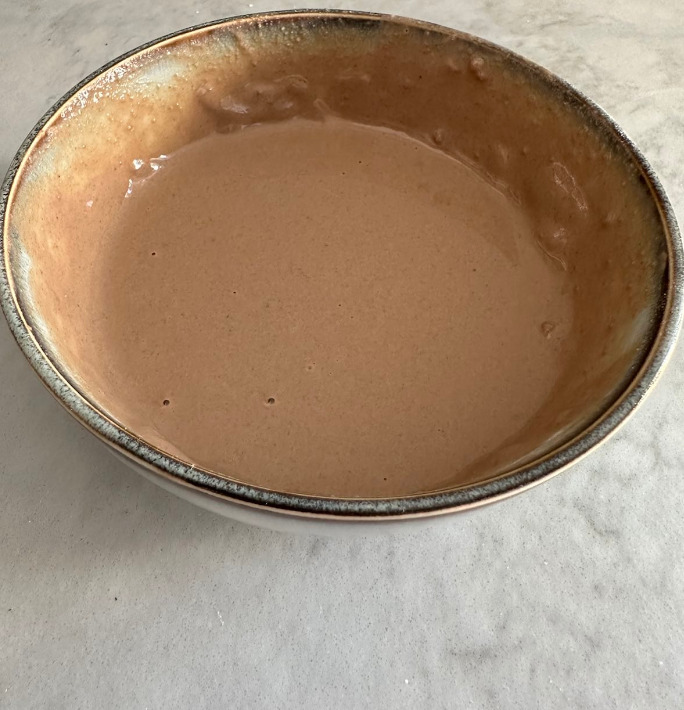
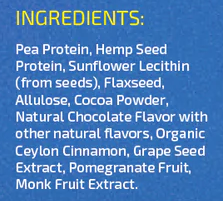
- mostly plant protein, giving you 26g of protein per serving
- allulose as a sweetener is great and I’m happy to see it becoming more common (see my post on allulose for why). may want to note to consumers what it does and why they might notice it
- cinnamon and grape seed both great inclusions
- no opinion on pomegranate and monk fruit extract. I’m assuming the latter is as a sweetener and flavor enhancer (BP: pomegranite included to improve metabolic panel markers)
- the taste is… alright. some people definitely like it more than I do, but I’d probably only rate it a 5/10 myself. I substituted whole milk in instead of nut milk as suggested and added the blueberry mix (below) to it to finish it. it tastes like a rather bland pudding, but a bit more dense and with little sugar or fat (BP: adding EVOO for taste may help)
Blueberry mix
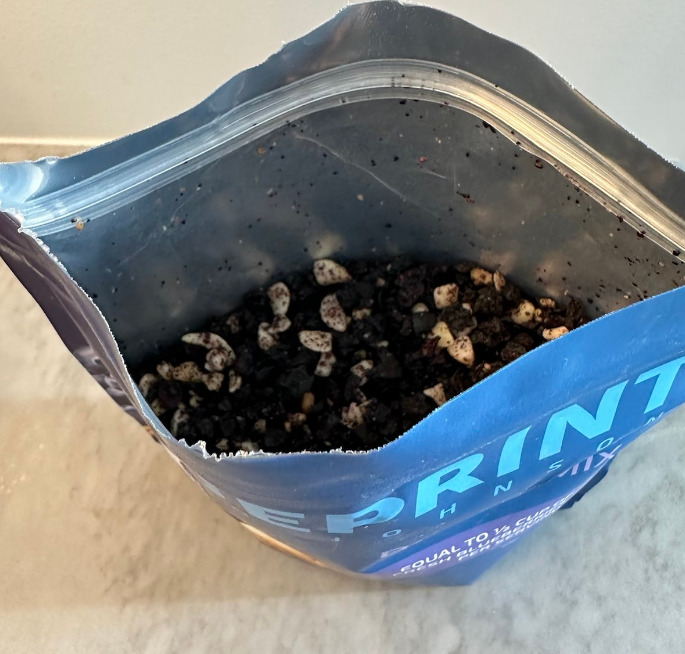
- it’s literally just dried blueberries, macadamia nuts, and walnuts
- tastes great no matter what do you with it really. I’d suggest adding it to the above pudding or combining it with some milk
- blueberries are great for you and a good snack to have around
- with that said, this mix is so simple that I’m not sure I gain much from having someone else prepare it for me (BP: mix replaces 2kg of berries and helps not having to acquire them frequently)
Longevity mix
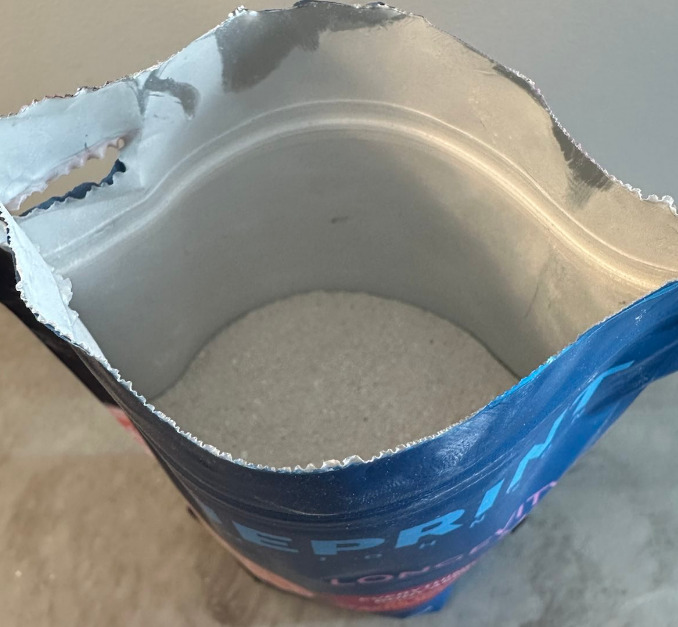
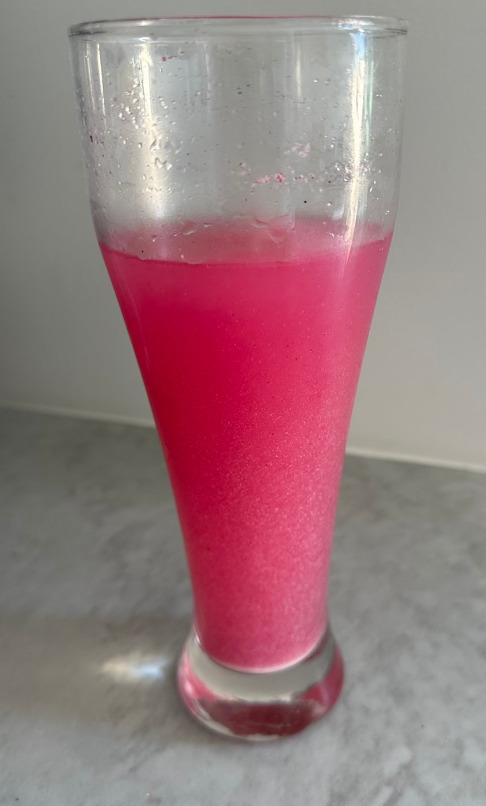
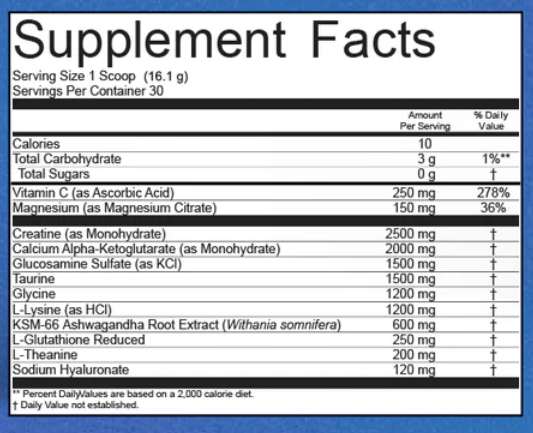
- simple mix that you add to water
- creatine, glucosamine, taurine, glycine, the gang’s all here
- allulose again chosen as the primary sweetener
- I’d increase the amount of glycine (1.5g), especially due to this being earlier in the day so it’s unlikely to be too much to disrupt sleep (this dose would be fine before bed and likely benefit sleep but the instructions online suggest to have this mix in the morning). for more information on why I’m in favor of macrodosing glycine check out the glycine section on my supplements page (hint: it extends lifespan in mice!)
- sodium hyaluronate is an interesting choice and I wonder why they chose it + this form of it (BP: intended to reduce inflammation and restore HA levels which decline with age)
- i’m a big fan of ashwagandha, with the note that it can cause digestive distress for some. great for lipid profile and often improves anxiety too
- there could be a bit more creatine (2.5g) for those lifting, especially since calcium alpha-ketoglutarate was added (BP: amount chosen to be tolerable for mass market while covering omnivores average diet intake)
- the taste is decent and comparable to most ‘vitamin’ drink mixes that you add to water. I’d probably rate it a 6.5/10, nothing to complain about but not particularly exciting
Pills!
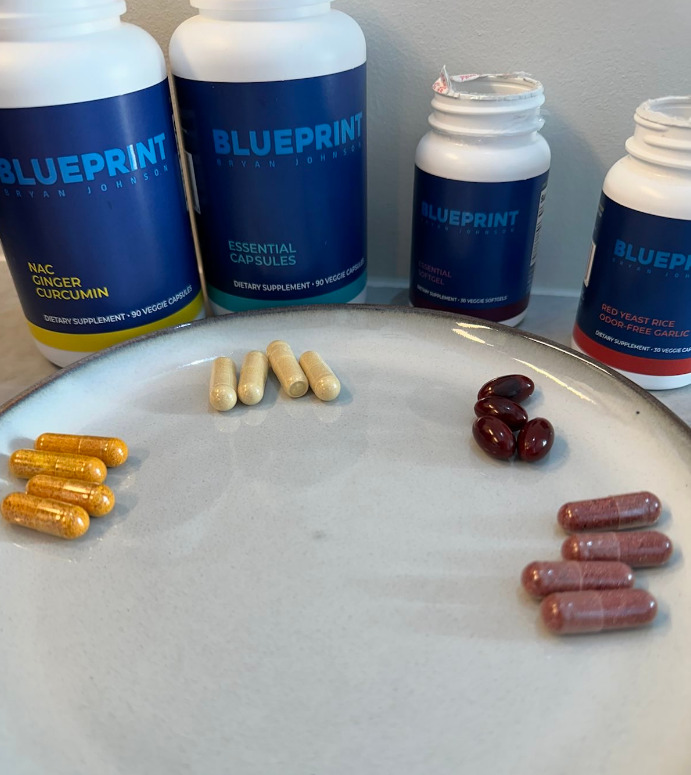
NAC, ginger, curcumin
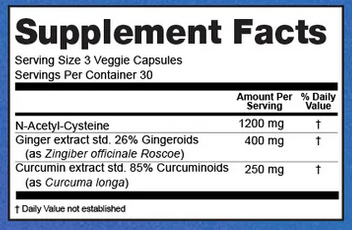
- NAC is reasonably popular in the longevity community and pretty interesting
- not 100% sure what the reasoning behind ginger was – I wonder if it was due to its ability to ameliorate potential gastrointestinal side effects of other supplements? (BP: intention is to improve metabolic panel, reduce inflammation, improve mood)
- circumin is generally a good choice, although I have concerns about lower bioavailability here. does one of the other supplements included notably modify this? (BP: curcumin bioavailability 4x higher in this case due to being bio-enhanced)
Essential capsules
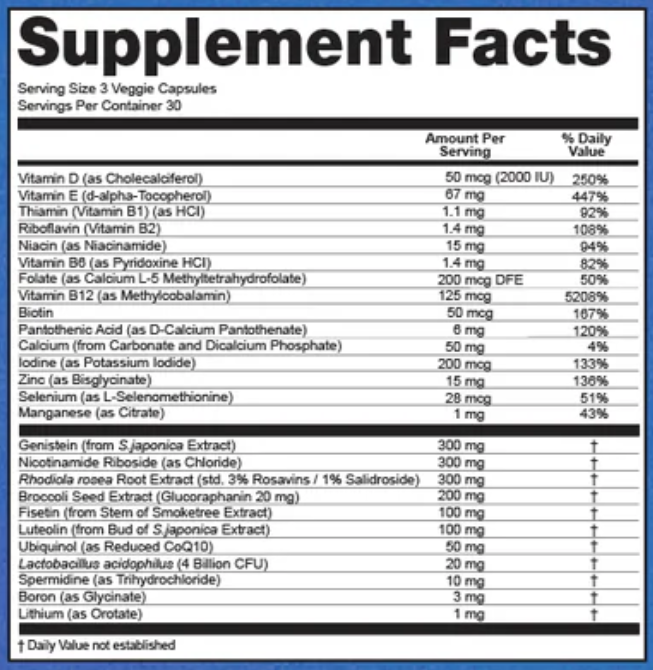
- not going to list all the vitamins as they’re all reasonable choices
- glad to see a higher amount of vitamin D (2,000 IU). could go even higher but the average blueprint consumer probably gets more sunlight than I do
- I don’t know what the benefits of including e.g. iodine, calcium, manganese, and selenium are. would these be missed at all if excluded? feel free to enlighten me if you’re a reader! (BP: these deficienties are moderately common so worth including, calcium as excipient of CaAKG)
- lithium is interesting at 1mg but I’m not sure that’s high enough to do very much (although perhaps this is the intention to keep it on the safe side)
- I didn’t know that the body converts glucoraphanin into sulforaphane via myrosinase. good supplement!
- fisetin at100mg daily is an interesting choice. curious how they decided daily was the correct increment and 100mg was the correct dose – I found this one hard to decide on myself (BP: dosage most studied wrt fisetin)
- spermidine 10mg is perhaps one of the most promising supplements here due to directly improving lifespan in mice! how did they source this? see more info in the spermadine section on my supplements page
- genistein is an interesting choice as it is pretty estrogenic. there are a lot of gender-ambiguous benefits to this, but i’m curious what the specific reasoning was and how the dose of 300mg was decided. it has affinity for α estrogen receptors, but not as much as β. if it was easier to source and include 17α-estradiol, would that have been included instead? was this included for entirely different reasons, of which there could be many? see also: does 17α-estradiol/estrogen extend male human lifespan? (BP: genistein does not increase blood estrogen levels in men, 17α generally requires Rx)
Essential softgel
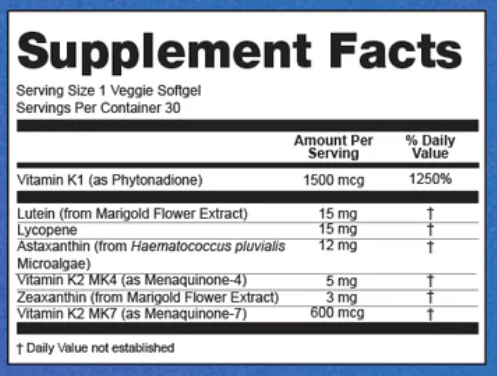
- nothing particularly exciting here, nice to see the better forms of vitamin k (k1, k2 mk4, k2 mk7) all included
- astaxanthin is a nice inclusion
- less excited over lycopene, lutein, and zeaxanthin, but I don’t know anything bad about them. would like to research them more later
Garlic, red yeast rice
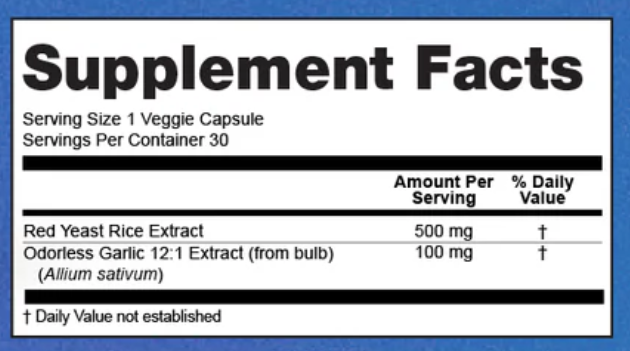
- unsure why these are in their own pill instead of included within one of the larger mixes (from which you take 3 daily from)
(BP: pills were grouped to reduce amount. water soluable actives went into longevity RTM, fat soluble into softgel, bitter tasting into capsules, and small amounts into essentual. RYR and NAC were separate SKUs due to regulatory concerns. - garlic is great if we give them the benefit of the doubt on the 12:1 exact including enough allicin
- does the red yeast rice (500mg) actually contain a lot of monacolin k? some countries like the US dislike this due to it being bioidentical to e.g. lovastatin (BP: no, this is why CoAs are shared)
- interesting side note: when you research a lot of natural compounds (roots, vegetable extracts, etc), it’s very interesting how many of them are bioidentical to actual pharmaceuticals. when I was young I didn’t understand why ‘vegetables’ or such should be good for me: adults would tell me they had “vitamins and minerals” in them, but I could obviously both a) test myself for vitamin and mineral deficiencies and b) supplement them if I was deficient in them. it wasn’t until many, many years later that I learned how amazingly complex everything we eat is and how many biologically active ingredients are in dishes. think of a dish with many vegetables, spices, roots, meat, etc, as basically having 20 different drugs in it, but with all of them in very small doses. many of them are also very slightly psychoactive too! just as you can get high on nutmeg and sweet potatoes inhibit α-glucosidase and are thus anti-diabetic like the drug acarbose and red yeast rice is literally the same as a statin medication and berberine found in plants like barberry is a mimetic of the anti-diabetic drug metformin, the foods we eat on a daily basis have an astoundingly large amount of downstream effects in the body which have nothing to do with the vitamins or minerals in them. the cases we tend to know about like marajuana or opium are not rare in that they have strong biological effects, but rather are only rare in the dose response curves that are common with consumption. the present epistemic milieu we reside in with respect to nutrition doesn’t talk about this much primarily because we know so little about the topic and actually learning what is going on enough to have high confidence and rigor is extremely tedious and slow
Olive oil
- it’s olive oil. it is made from olives
- the branding of ‘snake oil‘ is honestly pretty funny given how most supplement marketing is basically fraudulent
Marketing
- no cards or instructions were included in the box. this is missing out on a gigantic free lunch!
- I’d strongly suggest adding instructions, a thank-you card, and a discount code for future purchases
- the discount code should be notable as user retention may be more challenging than other monthly supplements due to the tediousness of consumption (8 pills, one drink mix, one pudding mix, olive oil, and another mix, every single day!)
- given the higher price point it would be great if the bags could be redone to have a high-quality zipper on them as sealing them is annoying
- the supplement bottles should be shinier. this will increase conversion as this product is offered at a ‘premium’ price point. I’d look into making a bespoke logo for blueprint as well or otherwise refining the font and decor around it. you will be surprised how much of a return you can get by spending $0.10 more on shiny paper and branding!
- I would move supplements with the highest probability of causing digestive distress into their own pill so that users can modulate dosage or ablate it themselves for e.g. supplements like ashwaganda and curcumin. this should be included in the instructions as otherwise users who feel unwell may simply never try blueprint again
- current marketing paradigm likely favors a cohort of more males than females and more adolescents than the elderly. this is reasonable and likely the correct choice to make early on, but I’d consider long-term strategies on how to improve this. encouraging gifting to the elderly (e.g. one’s parents) is likely a great idea here for obvious reasons
- (BP: agreed on much of the above, some are already being implemented)
Research and sourcing
- most of what is included is good, but I’m not told why it was included! if there was so much money spent on research and reviewing studies, it would be great if some of it could be shared to me as a lowly and uninformed end-user
- why does the blueprint page advertise ‘non-gmo’, and what does ‘no artificial ingredients’ mean? generally the latter means next to nothing, but I don’t actually care if something as simple to synthesize as e.g. glycine is ‘artificial’ or not as long as it is the right chemical and without contaminants or impurities! modify labels for your intended audience accordingly
- similarly to sharing research the website states that you “test the ingredients” yourselves. I assume this means more than just eating them and making sure you don’t die, but this seems like a great thing to share more about, especially because this is very tedious to do (BP: manufacturers are asked for CoA and then we test the individual ngredients ourselves)
- having users share metrics before and after a few months of blueprint consumption would be great (BJ told me isn’t necessary as the individual ingredients already have sufficient evidence on their own. but if you ask me, there is never enough science being done and there is never enough data, so I’d prefer we continue to learn all that we can even if it only confirms our priors) (BP: a few thousand people are on it and sharing biomarkers, we will share this data)
- relatedly I’m concerned that some of the supplements may inhibit various enzymes that then cause unknown and/or undesired changes of bioavailability in other supplements or drugs. both curcumin and ginger inhibit CYP3A4 and this may be suboptimal if combined with other longevity drugs like rapamycin (which, similarly, could block astaxanthin which blueprint includes as well). this is challenging as we have limited knowledge on this topic for most supplements and drugs, but is worth at least being aware of
- fortunately i’ve written from-scratch personal tracking software that I can throw all of this data into in order to solve a few of the questions (those which are easily answerable via blood tests at least), but this is costly and tedious and I am tired of getting blood tests. I may try A/B testing all of blueprint RCT-style to see if I can notice any other variables it effects that I wouldn’t have otherwise predicted
Final thoughts
- overall this is a decent purchase if you want to make the trade-off of spending more money for not having to perform your own research, logistics, sourcing, purchasing, etc
- with that said, there’s secondary effects to note: a) the substitution effect of having something decent rather than what you’d have had instead (this is why most ‘diets’ are always an improvement: the typical American diet is so bad that basically anything that is not insane is likely to be a net-benefit), and b) the psychological effect of causing you to consciously think more about optimizing for your health. I would strongly bet that there is a ‘healthy user bias’ among blueprint users that results in them having e.g. better lipid and glycemic profiles than non-blueprint users even if they stopped taking blueprint as they probably get more exercise than average
- the largest benefits in longevity may still be things that we cannot easily sell in a bag or bottle, whether that is because it is intangible like exercise, or because of excessive regulation (e.g. the revolutionary weight-loss drug semaglutide requires a prescription and often insurance, although notably can also be purchased online regardless from parties which are not fans of the US patent system. even so, clinical trials for it began 15 years ago which likely resulted in the early death of millions of americans due to obesity. other longevity drugs like rapamycin are also challenging to distribute to the average consumer for similar reasons (and that one is FDA-approved too!). the FDA is excessively conservative in what they allow, with drugs requiring a decade and ~$600M to be later slowly distributed to the general public, and this is before we even bring in talk of gene therapies or anything newer
- alpha in longevity (both personal and scientific) still remains in copious quantities for those who are willing to search for it!
- I haven’t yet decided if I’ll purchase another month of blueprint. I’ll try it for a bit longer and see how I feel, but I think it’s a reasonable product overall and I’m glad it exists
- If you enjoyed this post you might like my website (see also: supplements) or my twitter
- feedback (especially any potential corrections) is very welcome! DM me on twitter or submit anonymously here